Demystifying generative AI
Q&A with Graham Glass, CEO and Founder of CYPHER Learning
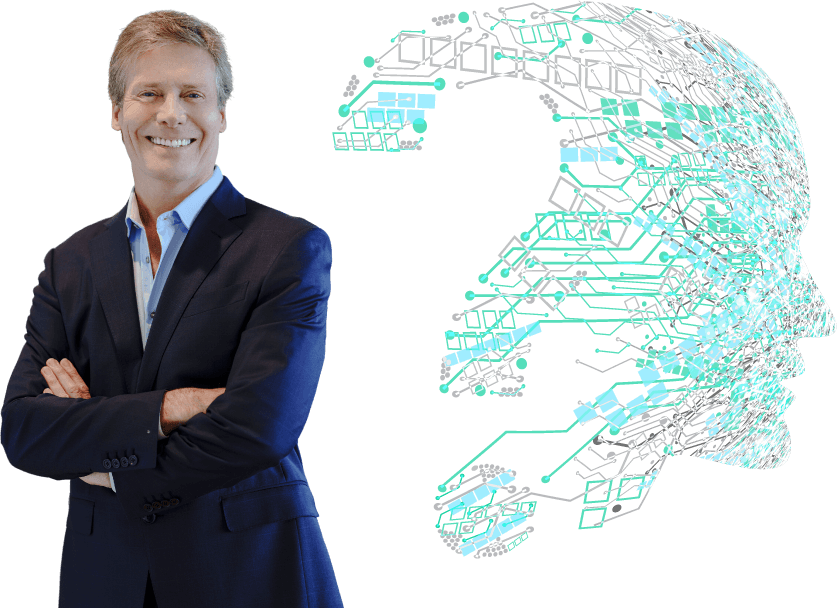
Demystifying generative AI
Amidst the whirlwind of artificial intelligence (AI) discourse, ranging from enthusiasm to caution, the narrative often misses a crucial point: AI's transformative power for training professionals. AI is not just another tool in the arsenal—it's a game-changer, enabling corporate trainers to quickly roll out tailored, competency-based skills development programs. This capability is revolutionizing learning and development (L&D), turning it into a key strategy for retaining talent and adding value to organizations across various sectors.
Graham Glass, the CEO and Founder of CYPHER Learning, is set to shed light on the immense potential of AI to customize and enhance learning experiences. By introducing foundational AI models like GPT-4 and DALL-E, Glass aims to demystify how AI can be leveraged in educational settings. A significant focus will be on the critical role of prompts, which are instrumental in maximizing the value derived from AI technologies. Readers can expect to gain insights into best practices for creating pedagogically sound and relevant content, illustrating how AI can be effectively integrated into educational strategies to meet the evolving needs of the modern workforce.
You asked. Graham answered.
Rewinding the clock by over three decades, there was burgeoning interest in an emerging technology known as neural networks. In their nascent stage, these rudimentary models—inspired by our understanding of the human brain's functioning—were employed to tackle elementary tasks. For instance, consider the task of predicting the next letter in a sequence when presented with the fragment "DO"; an early neural network might deduce that the ensuing letter completes the word "dog". Initially, these networks comprised a modest assembly of neurons, perhaps around 10 to 20, tasked with such sequence prediction. Although seemingly trivial, these early experiments laid the groundwork for more complex applications. As researchers expanded their ambitions, they began to challenge neural networks with more sophisticated tasks, such as completing the phrase "today is a sunny ____." Over time, these networks evolved to predict with increasing accuracy, identifying the missing word as "day." This progression marked the foundational steps towards the intricate neural network models we see today, capable of far more complex and nuanced tasks.
Over time, researchers began to expand the capabilities and applications of neural networks, although they initially encountered significant obstacles. The computational power available was insufficient, and technological limitations led to a period of stagnation, causing many to view artificial intelligence as a marginal field with limited potential. Despite these challenges, a dedicated group of pioneers persisted. With the advent of more powerful computing resources, they ventured into experiments with substantially larger neural networks. These enthusiasts proposed models comprising tens of thousands of neurons, discovering that the networks' performance improved as they scaled. This upward trajectory continued until they reached architectures with millions of neurons, at which point the neural networks began demonstrating remarkable abilities. They started training these expansive networks using a vast array of publicly available texts, such as the classic novel "War and Peace," marking a significant breakthrough in the field.
Researchers began by posing simple questions to the neural networks, such as their thoughts on specific chapters, and were initially surprised by the coherent responses they received. However, the excitement was tempered by the networks' tendency to veer into nonsensical outputs. For instance, a network might begin by thoughtfully commenting on "War and Peace," only to abruptly shift to irrelevant observations about the weather or express confusion about its purpose. This inconsistency highlighted the limitations of early neural networks, yet it did not deter the researchers. Persistence in the face of these challenges led to a significant breakthrough with the publication of a seminal paper titled "Attention is All You Need." This work introduced an innovative approach that significantly improved the AI's ability to maintain focus on relevant information, thereby reducing the occurrence of irrelevant or nonsensical responses.
The pivotal advancement in AI technology came with the development of the Transformer model, a breakthrough that significantly enhanced the capabilities of artificial intelligence systems, including those used in conversational AI like GPT (Generative Pre-trained Transformer). Over three decades, researchers faced numerous challenges, overcoming each to reach new levels of innovation. Approximately a year and a half ago, this iterative process culminated in the release of GPT-3, marking a watershed moment for AI interaction. For the first time, individuals could engage in meaningful conversations with an AI, receiving intelligent and coherent responses.
Many were skeptical at first, myself included. Initial tests with basic questions like "What's two plus two?" quickly evolved into more complex inquiries, such as drafting a course outline for digital marketing—a task GPT-3 performed with remarkable acumen. This experience was eye-opening, revealing the technology's potential to revolutionize how we approach education and learning.
Currently, the pace of development is accelerating. With the introduction of GPT-3.5, GPT-4, and the anticipation of GPT-5, alongside Google's Gemini 1.5, we are witnessing rapid advancements in AI capabilities. This progress is a testament to the persistent efforts of researchers and developers over the years, and we are now beginning to reap the benefits of their labor. For those of us in the education sector, this presents an unprecedented opportunity to leverage AI technology to enhance teaching and learning methods, making this an incredibly exciting time to be involved in the field.
A common curiosity revolves around whether, when prompted with a question, the AI merely regurgitates information it has encountered online, or why it doesn't provide direct links to its sources. These are valid inquiries that highlight a fundamental aspect of AI learning, which is somewhat parallel to human learning processes.
Consider GPT-4 as an example; it was trained on a dataset comprising 570 gigabytes of text, embodying a voracious reader absorbing vast quantities of information. However, unlike a simple copy-paste mechanism, it doesn't memorize data verbatim. Instead, the AI identifies patterns within the data, modifying the connections among neurons within its extensive neural network to encapsulate the core of what it learns. This involves distinguishing specific words, the relationships between words, and even grammatical structures observed in language.
It's noteworthy that GPT's training encompassed nearly every language known to us, making it a polyglot entity. This means you can interact with it in various languages, from Chinese to Swedish, and receive responses in the language of your inquiry. The AI's learning process involves crafting a web of connections that inform its understanding and responses, a testament to its intricate design and the sophistication behind its apparent simplicity.
GPT technology boasts over 1 trillion synaptic connections, a staggering number, though still dwarfed by the human brain's approximately 100 trillion. This comparison highlights our current edge over AI in terms of complexity, yet this gap may not persist indefinitely. Like humans, GPT learns by absorbing and repeatedly processing information to forge pathways of understanding, without retaining specific excerpts of text. Thus, when prompted with a question, GPT's response mirrors a human's: it synthesizes a novel sentence reflecting the accumulated essence of its training, rather than reproducing text verbatim from prior reading.
This process underscores two key points: firstly, AI generates original responses based on its "experiences," and secondly, it typically doesn't track the origins of its knowledge, mirroring human tendencies to forget precise sources of information. The absence of direct citation in AI responses is akin to the way most people recall facts—broadly, without specific details about the source.
In addition, part of AI's development involves reinforcement learning, where it interacts with humans to refine its capabilities, striving to meet our expectations. This effort to please can lead to AI attempting to provide answers or links without concrete knowledge, akin to how children might respond to questions with a mix of accurate and imaginative replies. While this eagerness to satisfy queries can result in inaccuracies, such as non-existent links, it's a reflection of the AI's learning process and its continuous improvement in delivering reliable information.
Hallucinations in AI responses are essentially the system's efforts to fulfill your queries satisfactorily. When the AI has ample information accessible, it tends to provide accurate answers. However, its performance may falter with questions concerning less common knowledge. For instance, as someone who frequently interacts with AI and has a fondness for the British sci-fi cartoon "Thunderbirds," I've noticed that the AI reliably answers questions about the show's main characters and spacecraft, reflecting the abundance of available data on these topics. Yet, when I inquired about a specific episode featuring a unique plot twist—a detail not widely documented—the AI inaccurately described the twist. This experience illustrates that AI is more prone to inaccuracies when dealing with obscure or scantily documented subjects, attempting to generate a response that, while intended to be helpful, might not be correct.
When engaging with AI, it's prudent to approach it with the same caution you would when interacting with another person: critically evaluate the information provided rather than accepting it unquestioningly. As we delve deeper into AI capabilities, it's crucial to consider the trajectory of this technology. I frequently consume content from leading figures in the AI field, such as Demis Hassabis of Google DeepMind and Sam Altman of OpenAI, who consistently convey a thought-provoking message: the current state of AI, as represented by models like GPT-4, is only the beginning. These systems are continually evolving, becoming more sophisticated, reliable, faster, and cost-effective. Significantly, their ability to reason and understand complex causal relationships is advancing.
For instance, while GPT-3 had limited reasoning capabilities, GPT-4 demonstrates a marked improvement in processing complex problems and deducing causality. This trend is expected to continue with GPT-5 and beyond. Visionaries like Hassabis anticipate that AI will not only enhance our capacity for innovation, including breakthroughs in medicine, aging, and even theoretical physics, but also transform the way we learn and solve problems. The consensus among experts is optimistic: AI will serve as a powerful ally in humanity's quest for knowledge and discovery, opening doors to possibilities that today seem like science fiction.
Before we explore AI in action, let's consider the user experience of engaging with AI technologies like GPT-4. Currently, text remains the dominant mode of interaction, though many users have begun experimenting with voice commands, finding them remarkably effective. Furthermore, the advent of vision capabilities has led some early adopters to monitor their emotional states via camera during interactions, enabling the AI to assess and respond to their feelings, such as happiness or sadness. This shift towards multimodal interactions—incorporating vision, speech, and text—suggests a future where AI engagement is more holistic.
However, the essence of utilizing AI lies in the interaction itself, which is more akin to a dialogue than a mere search. Engaging with AI is conversational by nature; you pose a question, receive a response, and may seek further clarification or a different perspective. This dynamic is crucial for maximizing the benefits of AI, particularly in fields like education where the initial focus has been on enhancing the efficiency and quality of content creation.
Interacting with AI, in my experience, is akin to engaging with a highly intelligent but occasionally distracted teenager. You might pose a question and receive an insightful answer initially, only to be met with a less relevant response upon further inquiry. This inconsistency suggests the need for more precise instructions. For instance, rather than broadly requesting a course outline, specifying the need for titles and descriptions of 10 course modules often yields a more focused reply. However, responses can vary in length or detail, prompting further refinement of the prompt to achieve the desired specificity, such as requesting descriptions that span two to three sentences for each module.
Despite these adjustments, the AI might still deviate from the request, delivering eight modules instead of the specified ten, demonstrating its occasional lack of precision. Persistently rephrasing or repeating the prompt is sometimes necessary to guide the AI towards the exact requirements. This iterative process of narrowing down the AI's response options mirrors the experience of gently coaxing a teenager to maintain focus and not get distracted.
Moreover, as we transition to newer, more advanced AI models, each comes with its unique set of characteristics. Adapting to these nuances is part of the learning curve, illustrating that effective communication with AI requires patience, specificity, and an understanding that it may have its own "personality quirks," much like getting a teenager to concentrate on the task at hand.
Our primary objective with AI integration was to streamline the process for educators to produce superior educational materials. As we continue to explore more sophisticated applications of AI, it's essential to understand the types of queries that elicit the most valuable responses. This involves leveraging multiple AI systems, not just one, to achieve desired outcomes, emphasizing the importance of thoughtful interaction in unlocking AI's full potential.
Navigating AI's peculiarities necessitates a period of adjustment to optimize interaction. In the context of education, our objective was to streamline the AI utilization process, eliminating the need for end-users to master complex prompt engineering. We achieved this by developing and refining a series of prompts over several months, then concealing this complexity behind simple, user-friendly interfaces. Users can now issue straightforward commands, and the AI executes these tasks seamlessly on their behalf. This approach reflects a broader trend towards simplifying AI interactions, ensuring users can harness the technology's benefits without becoming experts in prompt formulation.
An especially intriguing discovery was AI's capacity for supporting internationalization effortlessly. Initially, the assumption was that prompts needed to be translated into the target language for the AI to generate responses accordingly. For instance, creating a course in English implies using English prompts, with the expectation that translating these prompts into Spanish would be necessary for Spanish content creation. However, to my surprise and relief, it was found that one could simply instruct the AI in English to produce responses in another language, whether it be Spanish, Swedish, or Swahili. This revelation significantly reduced the anticipated workload and opened up exciting possibilities for multilingual content creation, demonstrating AI's remarkable flexibility and adaptability in catering to diverse linguistic requirements.
Discovering the innate multilingual capabilities of AI was a moment of profound realization for me. Initially, I wondered if the technology relied on external services like Google Translate for language translation, assuming the AI processed everything in English before converting the output. However, the truth is far more impressive: AI systems inherently understand multiple languages. When prompted in Spanish, for example, the AI doesn't merely translate from English; it comprehends and applies Spanish grammar directly, conceptualizing the response in the language requested. This revelation underscored the AI's sophisticated grasp of diverse languages, a feature that genuinely astonished me.
Acknowledging the questions and engagement from the audience, I am eager to demonstrate AI's capabilities firsthand, believing strongly in the power of direct experience to convey the technology's revolutionary impact. While discussions about AI's potential are enlightening, nothing compares to witnessing its application, especially in creating educational content—a field where its impact is both immediate and transformative.
As we prepare to explore AI in action, it's important to note that multiple AI models may be utilized to achieve the desired outcomes, underscoring the technology's versatility. This approach is not about relying on a single AI to handle every task but leveraging the strengths of various models to enhance the educational experience. This exploration aims to showcase the practical applications of AI in education and hint at future possibilities beyond the initial phase of adoption, highlighting the technology's expansive potential.
AI technology encompasses a diverse range of specialized models, each excelling in specific domains: image synthesis, voice generation, content discovery, and text creation, among others. For instance, innovations in video synthesis, such as the early previews of sawa, highlight the rapid advancements in this field. This diversity means that multiple AI systems are often coordinated to achieve complex tasks, operating seamlessly behind the scenes.
For users, this integration offers a simplified experience, allowing them to leverage AI's power without needing to understand the underlying technology. The process I'm about to demonstrate involves coordinating hundreds of prompts in a dialogue with these AI systems, a task that is far too cumbersome to manage manually through platforms like ChatGPT. Instead, we employ parallel processing, engaging multiple AI models simultaneously to gather pieces of information, which are then assembled to form a cohesive whole.
The AI landscape is diverse, with specialized models excelling in specific areas: some shine in image synthesis, others in voice synthesis, still others in information retrieval, and many are adept at generating text. Innovations are continually emerging, such as sawa's preview indicating the near-future potential for video synthesis. This variety underscores the vast array of AI capabilities, all operating in concert behind the scenes.
A notable aspect of modern AI technology is its user-friendly accessibility. Individuals can harness the capabilities of AI effortlessly, without delving into the technical intricacies, due to the sophisticated integration of these systems into intuitive platforms.
This seamless operation involves coordinating a vast number of prompts in a dynamic exchange of information between the interface and various AI models. This coordination happens with exceptional speed and efficiency, allowing for a fluid interaction. By automating this complex dialogue, users are relieved from the laborious process of manually inputting each prompt, showcasing the advanced backend processes that facilitate a streamlined and effective user experience.
Utilizing AI technology often involves simultaneously engaging multiple AI models to integrate their distinct inputs into a cohesive outcome, akin to piecing together a jigsaw puzzle. This method utilizes publicly available data, notably the 570 gigabytes ingested by these models, ensuring a broad and up-to-date knowledge base as of December 2023. Although this may exclude the very latest updates, it underscores the substantial information these AIs can access. The future of AI promises even more dynamic capabilities, with advancements aimed at achieving real-time processing. This will significantly reduce the gap between real-world events and AI recognition. Moreover, the interest in creating educational content from private data highlights AI's flexibility and its potential to customize educational resources to meet diverse requirements.
Ensuring the accuracy of information provided by AI involves a combination of human expertise and critical thinking. As someone deeply versed in computer science and education, I can scrutinize the AI-generated content for precision, leveraging my knowledge to assess its correctness. This process is significantly more efficient than creating content from scratch, though it does require vigilance for potential inaccuracies.
When doubts arise about the AI's output, cross-referencing with additional AI models or conducting a quick online search can provide validation. This approach is not exclusive to interacting with AI; it mirrors the critical evaluation applied to any information source, given the prevalence of misinformation in various media.
To enhance reliability, we're developing a tool called AI Crosscheck, which employs a secondary AI from a different provider to review the initial AI's output. This method increases the likelihood of verifying the accuracy of the information, as agreement between two independent AI systems reduces the chance of propagating false information.
Ultimately, navigating the landscape of information, whether from AI or human sources, demands discernment and proactive verification to mitigate the impact of misinformation. Engaging with AI, in this regard, is akin to navigating any information-rich environment, necessitating a judicious approach to discerning truth from falsehood.
Clarifying concerns about data protection and intellectual property (IP) when utilizing platforms like CYPHER is essential, as these questions are common across many users and similar platforms, including Microsoft's Copilot.
The primary concern revolves around how user data is handled: “Is it stored, or could it potentially be exposed or utilized without permission?”
To address these concerns, it's important to note that platforms like ours do not store user data. Instead, we interact with external APIs provided by companies such as Microsoft, which are legally obligated to protect user data as per their terms of service. These companies assure that the data transmitted via their APIs is used solely for the purpose of responding to user queries and is not saved for any other use.
For users of free services, there might be different policies in place regarding data usage for training purposes. However, when engaging with paid API services, there are stringent guarantees that the data will not be retained or misused, backed by the provider's financial and legal incentives to uphold these privacy standards. Thus, any information sent through these APIs is processed securely and then discarded, with the resulting content—such as course material—remaining the sole property of the user, free from any claim of copyright by the platform. This system ensures a high level of protection for users' data and intellectual property.
Resources
Got a question about AI? Ask it here and we'll see what Graham says!